We’ve all experienced the hype around agentic AI, with companies eager to integrate the promise of artificial intelligence into every corner of their operations. However, many businesses are underestimating the complexity of actually making it work—rushing in without a real strategy and inadvertently setting themselves up for costly failures. Without the right data infrastructure to provide holistic, real-time context, AI-driven systems risk becoming an expensive way to scale bad experiences—it’s like turning a single frustrating call center experience into a million automated bad experiences. True success with agentic AI depends on much more than just plugging in a language model. It requires a comprehensive approach that ensures AI decisions are informed, relevant, and actually helpful for your customers.
Context Matters: The Key to Intelligent AI
AI doesn’t just need data—it needs the right context to make intelligent, informed decisions. A generic large language model (LLM) understands the world broadly, but that alone is not enough to create a useful AI system. For AI to be truly effective, it must be equipped with domain-specific knowledge, such as company policies, product details, and service guidelines. It also needs user-specific data, including purchases, support interactions, and behavioral patterns. Most critically, AI must be aware of the real-time state of things—current transactions, inventory levels, and other dynamic variables that affect the decision it needs to make at that moment. Without this full picture, AI is just an expensive tool making uninformed guesses.
There are a wealth of solid options for building the foundation of useful AI applications, which start with a foundational model, trained on vast amounts of general data. This provides a broad understanding of language and common knowledge, but it lacks the ability to personalize responses or make situationally aware decisions. Too many people seem to think they have everything they need at this point and are prepared to spend millions of dollars on input and output tokens, believing that it will pay for itself. But to actually make the responses relevant, businesses must layer in organization-specific data: information about the company, its products, policies, and how it operates. This retrieval-augmented generation (RAG) approach allows AI to move beyond generic responses and begin functioning as a domain expert, able to answer questions and perform tasks within a specific environment. But that’s just the first additional layer of context required.
The next critical layer is customer-specific history. Your agentic AI solutions must be able to recognize and adapt to individual users based on their past interactions. What has this customer bought before? Have they had support issues in the past? What are their preferences, concerns, or ongoing engagements? This information allows AI to provide personalized and relevant assistance instead of treating every user like a complete stranger. Without this, even an advanced AI is nothing more than a chatbot that repeatedly asks for the same information, frustrating users rather than helping them.
Finally, the most crucial piece is real-time awareness. No matter how much historical data and business context an AI has, it cannot make informed decisions and offer intelligent responses without knowing what’s happening in the moment. A customer might be attempting to complete a purchase, disputing a charge, or needing immediate support for a failed transaction. AI must be able to access and process real-time information—what the customer just tried to do, what system errors occurred, or whether their credit card was just declined. Without this layer, the AI is responding based on outdated assumptions, leading to responses that are not just unhelpful, but often actively misleading.
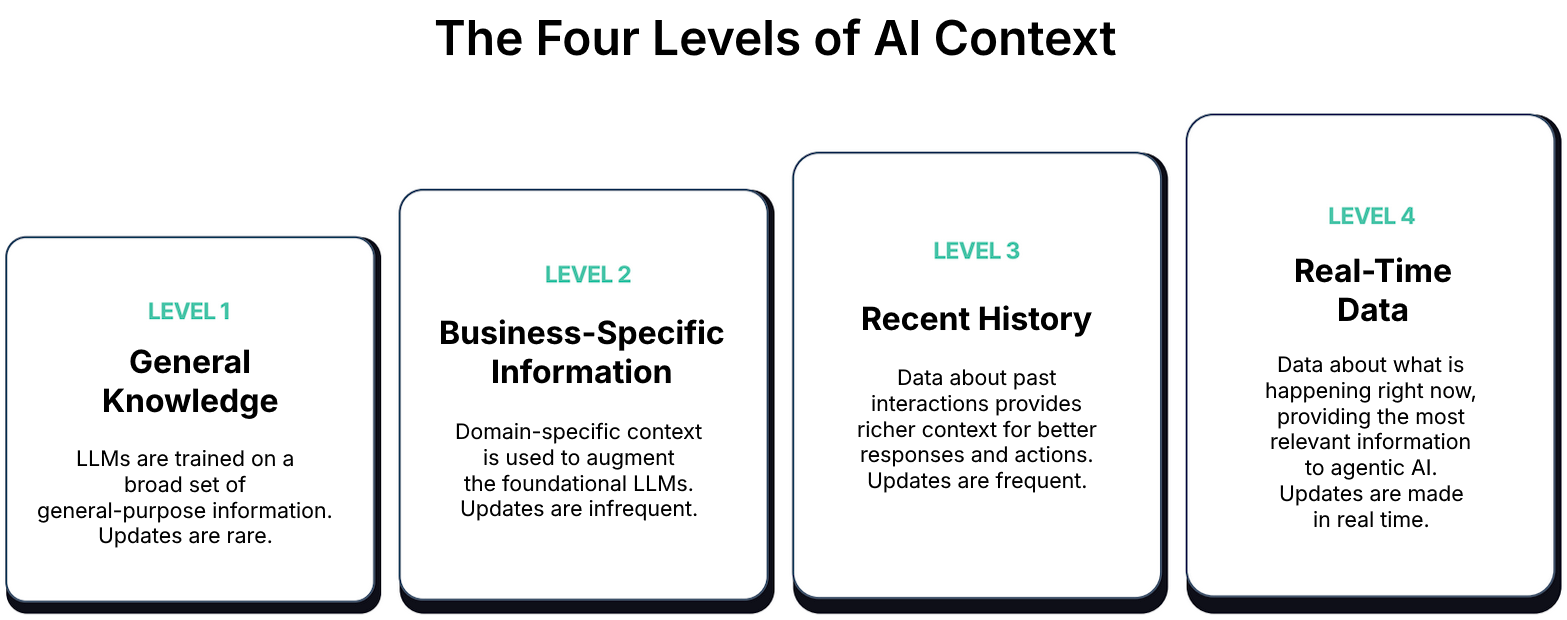
Without these layers of context—general knowledge, business-specific information, user history, and real-time data, your expensive AI app is just guessing. And most of the time, it guesses wrong. The false promise of AI is that it will magically improve experiences just by existing. But in reality, agentic AI is only as good as the data it operates on. A self-driving car trained on billions of miles of past driving data is useless if it can’t detect a pedestrian stepping into the street in real time. Similarly, an AI-powered customer service agent that lacks real-time transaction data is no better than an uninformed human agent giving generic, unhelpful responses. The success of AI isn’t just about having data—it’s about having the right data, at the right time, to make the right decisions and offer the right responses.
Avoiding the AI Hype Trap: A Practical Path Forward
Companies that treat AI as a quick-fix solution—just “smearing AI” on existing systems—will quickly realize that this leads to more problems than solutions. Instead, building a truly effective AI system requires a well-architected platform that integrates agent development, execution, and access to the best models, while also allowing for fine-tuning based on real-world interactions.
- Build a context-aware AI platform. Rather than relying on a generic LLM, companies must develop a platform that integrates agent development, model selection, fine-tuning, and real-time execution. This platform needs to enable retrieval-augmented generation to inject domain-specific knowledge, such as company policies and product details, and provide a framework for managing agent interactions to ensure AI responses are aligned with business goals and compliance requirements.
- Establish a real-time data pipeline. One of the biggest challenges in making AI truly useful is the complexity of integrating real-time data. Many businesses rely on batch processing, where data is collected, stored, and analyzed in periodic chunks. However, batch processing isn’t sufficient for AI-driven decision-making—by the time the data is processed, it’s already outdated. Your AI applications need continuous access to live, relevant information to make informed decisions.
- Integrate historical and session-based memory. An AI agent must remember not just long-term customer history but also the immediate context of a session. To achieve this, your architecture will need to store and retrieve a history of interactions, which could include data about past purchases, support interactions, IP addresses used in attempted fraud, etc.
- Prioritize reliability, scalability, and security. AI is not just another analytics tool—it must function as a mission-critical operational system. Success requires high availability (99.999% uptime) to support real-time decision-making without disruptions. In addition, enterprise-grade security and adherence to compliance requirements are critical to handle sensitive customer data responsibly.
- Test, iterate, and continuously improve. Deploying AI isn’t a one-time effort—it requires constant learning and refinement. Best practices include monitoring AI performance with metrics and feedback loops to evaluate how well the decisions, responses, and actions of your agentic AI are doing in the real world. It will be necessary to fine-tune models based on new data, emerging customer needs, and your evolving business strategies.
By following this structured approach, companies can build AI that genuinely enhances customer experiences rather than becoming an expensive failure. More importantly, it needs to be backed by the right data infrastructure to provide the necessary context for AI-driven decision-making. Without this foundation, AI initiatives will fall short, creating frustration, financial loss, compliance and safety issues, and missed opportunity for both businesses and customers. Companies that ignore this need will find themselves stuck in the "trough of disillusionment," where AI investments don’t deliver meaningful improvements and instead create inefficiencies, wasted resources, and exasperated users.
AI in production is less like traditional analytics (OLAP) and more like high-performance operational systems (OLTP). Unlike historical reporting tools, which can often tolerate a substantial amount of lag, AI-powered systems need to operate with near-zero latency, high availability, and the ability to make real-time decisions at scale. For example, a useful AI-powered chatbot on your favorite retailer’s website isn’t just answering static FAQs—it’s engaging with live customer transactions, helping users navigate purchasing decisions, troubleshooting order issues, and providing personalized recommendations. If this AI lacks real-time transactional data, it risks offering outdated or misleading information, damaging customer trust and impacting sales.
Supporting agentic AI at this level requires a sophisticated data infrastructure that can handle the speed, scale, and reliability demands of real-time interactions. Companies need robust data pipelines that continuously pull in operational signals, process them instantly, and feed AI agents with the freshest context. This isn’t just a technical challenge—it’s a fundamental shift in how businesses approach AI deployment. Those that invest in the right architecture will gain a significant competitive advantage, while those that cut corners or just deal with their current data infrastructure limitations will find themselves struggling with AI systems that simply create more problems than they solve.
AI’s Future Belongs to the Prepared
The future of AI belongs to those who are prepared to build it the right way. Agentic AI isn’t just another feature to bolt onto existing systems—it’s an entire shift that requires careful planning, robust data infrastructure, and real-time intelligence. The gap between AI success and failure is massive, and there’s little room for half-measures. Companies that get it right will set a new standard for intelligent, seamless customer experiences, while those that rush in without a solid foundation will find themselves wasting resources, alienating customers, and amplifying inefficiencies and existing challenges.
The real winners in AI will be those who understand that high-quality, real-time data is the key to unlocking AI’s full potential. A retailer that can instantly recognize its customers, track their purchase history, and provide seamless, real-time inventory and payment processing will create a frictionless shopping experience that drives loyalty and revenue. In contrast, a competitor relying on outdated or incomplete data will struggle with slow, inaccurate, and frustrating AI-driven interactions—leading to lost sales and declining customer trust.
Ultimately, the companies that dominate in AI will be the ones that invest in the right architecture from the start. This means prioritizing real-time data pipelines, context-aware AI, and systems designed for reliability and scale. The path forward is clear: AI leaders must move beyond the hype and focus on building intelligent, data-driven experiences that genuinely improve customer interactions. Those who do will pull ahead, while those who don’t risk falling into the AI trap—wasting millions on technology that does more harm than good.