Decodable attended the Data + AI Summit organized by Databricks in late June. With the announcement of the new Decodable Delta Lake connector at the Summit, Databricks users now can ingest data from any source on any cloud, simply and reliably. We value this partnership with Databricks and were excited to hear the announcements and keynotes, and to connect with the Databricks community. In this blog we highlight key announcements and insights from the conference.
Databricks did more than just unveil news at the Summit— although there was plenty of that. Through a series of keynotes, sessions and announcements, the company behind the data lakehouse made a strong case for how the center of gravity in big data analytics won’t be taken over by major cloud players any time soon.
Speed and Confidence
In the face of competition from their only distribution channel—the hyperscaler clouds—Databricks’ speed to market, execution and innovation means they outcompete native cloud offerings and now have the confidence to open source the majority of their previously commercial-only features and new capabilities. With new open source table storage layers coming onto the market - notably Apache Hudi and Apache Iceberg - this is a net-positive move for customers and keeps Delta Lake in the leading position.
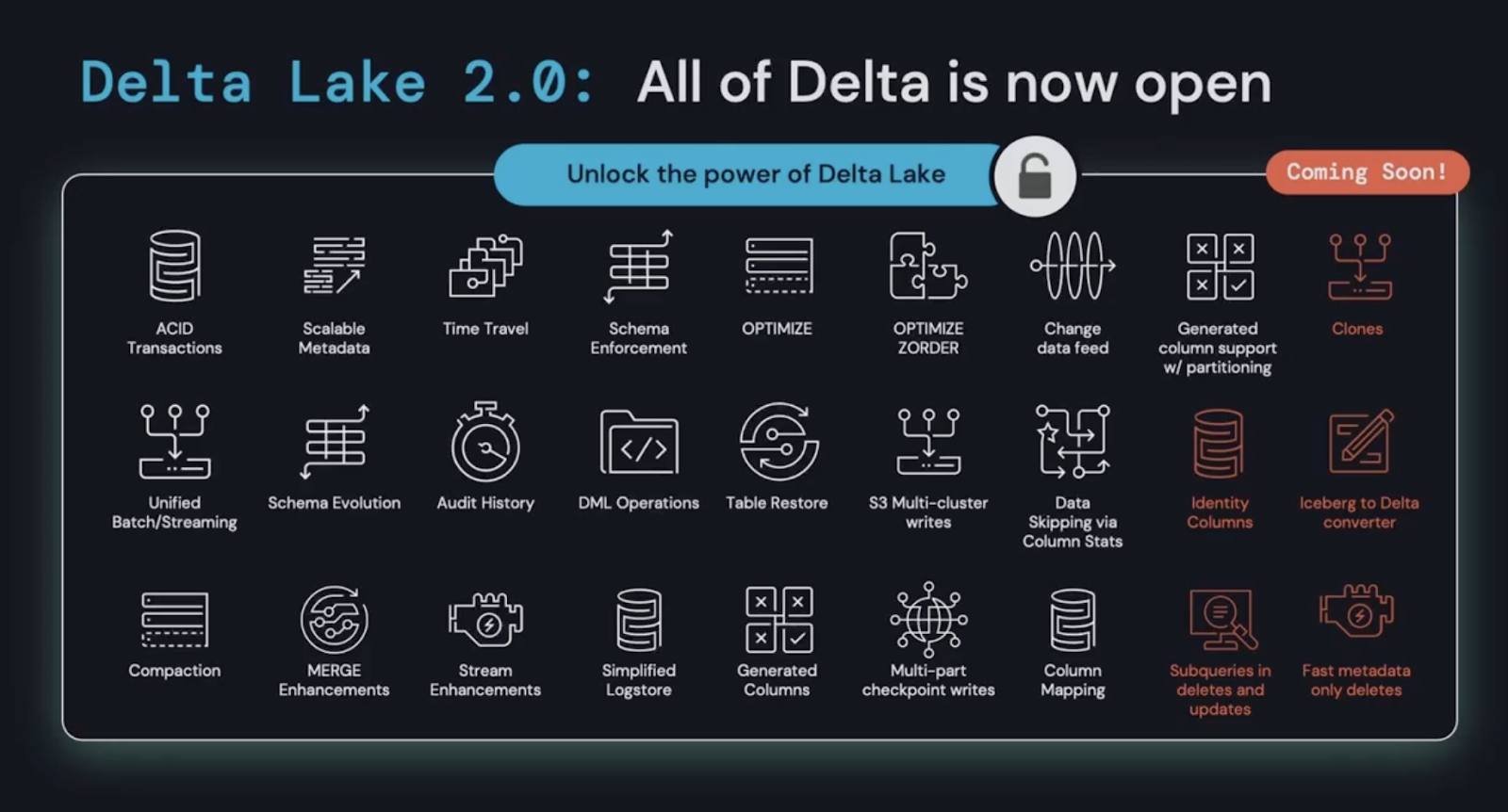
Jump onto the platform
Databricks is successfully navigating the transition to an independent data platform player with a growing partner ecosystem, reshaping and consolidating customers’ approaches to their traditional SQL-based data warehousing and emergent use of AI. Welcoming a broad range of partners—some of which compete with native capabilities—and making them available within product with the new “partner connect” integration, Databricks displays confidence and momentum in their core business to power them to the next stage of their evolution. It’s obvious that Databricks believes partners and a thriving ecosystem are going to drive the next phase of growth for the company.

Databricks now enables customers to publish their own data products on the newly announced Databricks Marketplace. Built on the open Delta Sharing standard, data consumers can discover datasets, notebooks, dashboards and machine learning models to accelerate building solutions on the platform. This further decreases the time to value for many use cases; another win for customers.

All paths lead to the Lakehouse
Databricks championed the movement to unify data warehouses and data lakes. Hyperscalers and other data vendors have already started wearing Databricks’ Lakehouse clothing to describe their own offerings, further cementing how this new category is being adopted by customers across industries. Databricks is building on its leadership position by creating new services atop the Lakehouse, a primary example of which is the newly available Unity Catalog for lakehouse management and data lineage.

In addition to announcing the GA of Unity Catalog, new features were added:
- Graphical Lineage visualization and interactive exploration
- Partner integrations, enabling Unity Catalog to extend across partner products.
- Search discovery capabilities
- SQL access to files and audit logs
Firing on all cylinders
Parallel innovation across all four key areas—Data Warehousing, Data Engineering, Data Streaming and Machine Learning—speaks to the scale and talent depth available in the engineering and product organizations, supporting the overall Lakehouse vision. It will be interesting to see if they can serve all of these use cases and audiences simultaneously with such an ambitious vision.
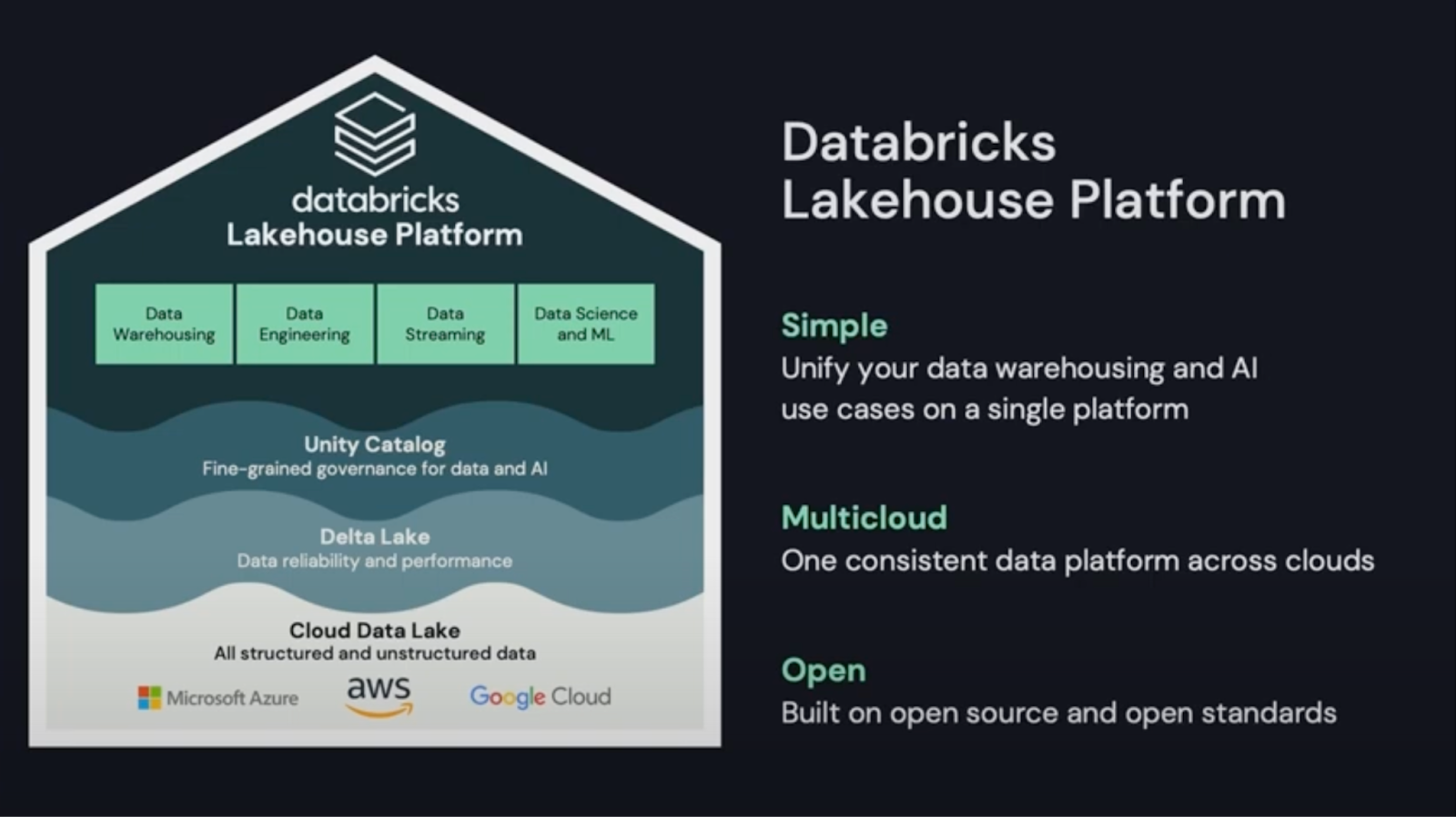
Data warehouse
A wealth of new features for data warehousing are now available including Photon, the fastest query engine for Lakehouses. Others include:
- SQL REST API, Go, Puthone & Node.js clients
- Python User Defined Functions
- Query Federation
- Materialized Views
- Data Modeling with Constraints
- Vectorized Sort
- Accelerated Window Functions
- Accelerated Parquet + Delta Scans

Data Engineering
Delta Live Tables is Databricks’ solution for getting data into Delta Lake. Alongside new features, Databricks announced it’s working on project Enzyme, a cost optimizer designed to select the most cost-effective ETL algorithm for the ETL job at hand.

Machine Learning & Data Science
For data scientists, Databricks’ MLFlow offering gets a 2.0 release which introduces the concept of pipelines: production-grade automated MLOps workflows.
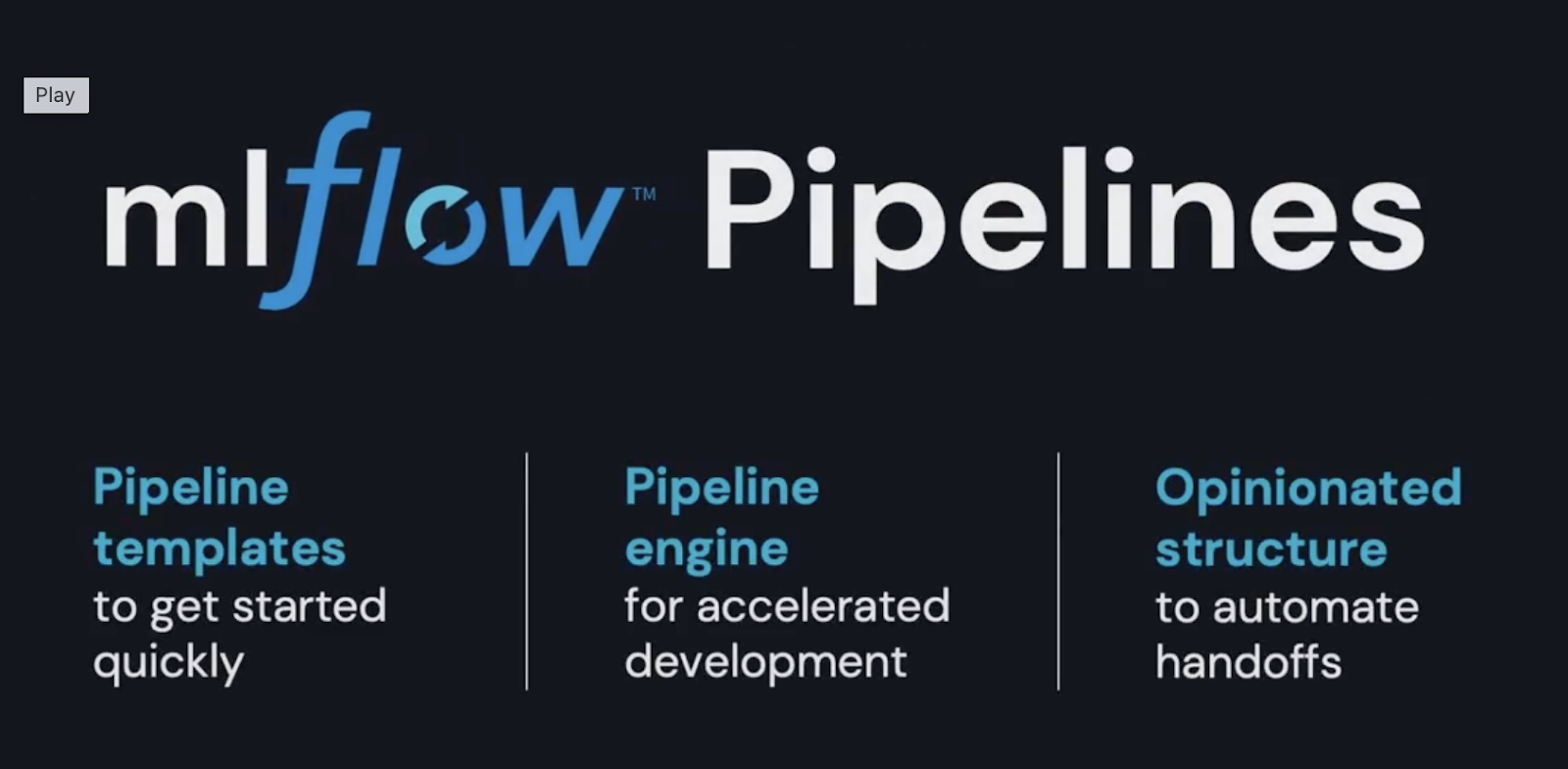
Spark
Spark burns ever brighter now, thanks to investments by Databricks and the community in the open source foundation. Incremental capabilities in Apache Spark version 3.2 and 3.3 were announced along with a new “Spark Connect” thin client with the promise of making Spark available everywhere, including edge devices, IDEs and backend servers.

Conclusion
Databricks had an opportunity to capitalize on the first in-person conference since the pandemic, and the company and community did not disappoint. They staked a claim as an independent platform with momentum, ecosystem and most importantly vision in data and execution to continue as a viable alternative to the hyperscale cloud providers’ native offerings.